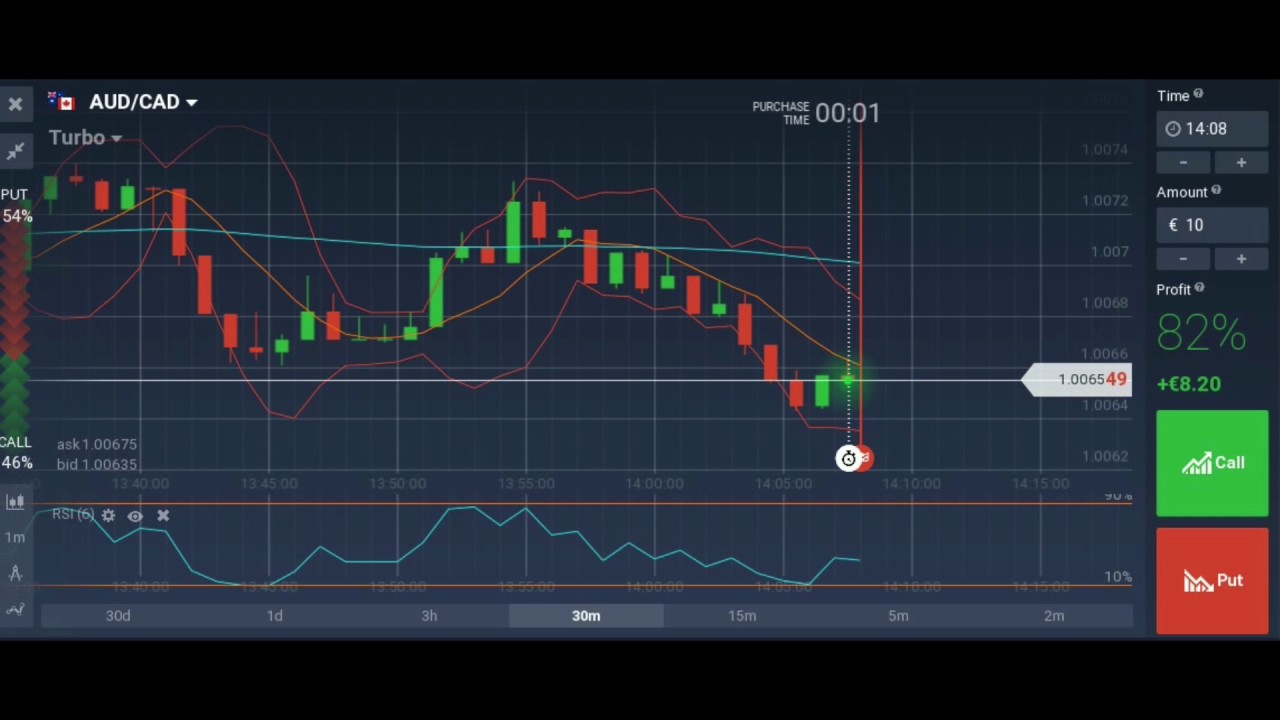
Exam Questions - Binary Numbers. Binary questions can come in a number of different forms. Reveal Answers. 8 (a) Question 8 part (a) may initially look like a bigger question than it actually is. The question explains that you have two registers which it uses to Binary test options (8 to 5) crossword clue. Posted on December 8, by jumble. Please find below all the Binary test options (8 to 5) crossword clue answers and solutions for the Universal Crossword December 8 Answers. In case something is wrong or missing kindly let me know and I will be more than happy to help you out with the right How to convert decimal to binary Conversion steps: Divide the number by 2. Get the integer quotient for the next iteration. Get the remainder for the binary digit. Repeat the steps until the quotient is equal to 0. Example #1. Convert 13 10 to binary
Binary Calculator
Binary classification is the task of classifying the elements of a set into two groups on the basis of a classification rule. Typical binary classification problems include:. Binary classification is dichotomization applied to a practical situation, binary test options 8 to 5. In many practical binary classification problems, the two groups are not symmetric, binary test options 8 to 5, and rather than overall accuracy, the relative proportion of different types of errors is of interest.
For example, in medical testing, detecting a disease when it is not present a false positive is considered differently from not detecting a disease when it is present a false negative. Statistical classification is a problem studied in machine learning. It is a type of supervised learninga method of machine learning where the categories are predefined, and is used to categorize new probabilistic observations into said categories.
When there are only two categories the problem is known as statistical binary classification. Each classifier is best in only a select domain based upon the number of binary test options 8 to 5, the dimensionality of the feature vectorthe noise in the data and many other factors. For example, random forests perform better than SVM classifiers for 3D point clouds.
There are many metrics that can be used to measure the performance of a classifier or predictor; different fields have different preferences for specific metrics due to different goals. In medicine sensitivity and specificity are often used, while in information retrieval precision and recall are preferred.
An important distinction is between metrics that are independent of how often each category occurs in the population the prevalenceand metrics that depend on the prevalence — both types are useful, but they have very different properties. Given a classification of a specific data set, there are four basic combinations of actual data category and assigned category: true positives TP correct positive assignmentstrue negatives TN correct negative assignmentsfalse positives FP incorrect positive assignmentsand false negatives FN incorrect negative assignments.
These can be arranged into a 2×2 contingency tablewith columns corresponding to actual value — condition positive or condition negative — and rows corresponding to classification value — test outcome positive or test outcome negative. There are eight basic ratios that one can compute from this table, which come in four complementary pairs each pair summing to 1. These are obtained by dividing each of the four numbers by the sum of its row or column, yielding eight numbers, which can be referred to generically in the form "true positive row ratio" or "false negative column ratio".
There are thus two pairs of column ratios and two pairs of row ratios, and one can summarize these with four numbers by choosing one ratio from each pair — the other four numbers are the complements. In diagnostic testing, the main ratios used are the true column ratios binary test options 8 to 5 true positive rate and true negative rate — where they are known as sensitivity and specificity.
In informational retrieval, the main ratios are the true positive ratios row and column — positive predictive value and true positive rate — where they are known as precision and recall. One can take ratios of a complementary pair of ratios, yielding four likelihood ratios two column ratio of ratios, two row ratio of ratios. This is primarily done for the column condition ratios, binary test options 8 to 5, yielding likelihood ratios in diagnostic testing, binary test options 8 to 5.
Taking the ratio of one of these groups of ratios yields a final ratio, the diagnostic odds ratio DOR. There are a number of other metrics, most simply the accuracy or Fraction Correct FCwhich measures the fraction of all instances that are correctly categorized; the complement is the Fraction Incorrect FiC.
The F-score combines precision and recall into one number via a choice of weighing, most simply equal weighing, as the balanced F-score F1 score. Some metrics come from regression coefficients : the markedness and the informednessand their geometric meanthe Matthews correlation coefficient.
Other metrics include Youden's J statisticthe uncertainty coefficientthe phi coefficientand Cohen's kappa. Tests whose results are of continuous values, such as most blood valuescan artificially be made binary by defining a cutoff valuewith test results being designated as positive or negative depending on whether the resultant value is higher or lower than the cutoff.
However, such conversion causes a loss of information, as the resultant binary classification does not tell how much above or below the cutoff a value is. As a result, when converting a continuous value that is close to the cutoff to a binary one, binary test options 8 to 5, the resultant positive or negative predictive value is generally higher than the predictive value given directly from the continuous value. In such cases, the designation of the test of being either positive or negative gives the appearance of an inappropriately high certainty, while the value is in fact in an interval of uncertainty.
On the other hand, binary test options 8 to 5, a test result very far from the cutoff generally has a resultant positive or negative predictive value that is lower than the predictive value given from the continuous value. From Wikipedia, the free encyclopedia. This article needs additional citations for verification. Please help improve this article by adding citations to reliable sources.
Unsourced material may be challenged and removed. Find sources: "Binary classification" — news · newspapers · books · scholar · JSTOR May Learn how and when to remove this template message.
Main article: Evaluation of binary classifiers. Mathematics portal. VIP Lab Publications. CiteSeerX Lu and C. Rasmussen Outline Index. Descriptive statistics. Variance Standard deviation Coefficient of variation Percentile Range Interquartile range. Central limit theorem Moments Skewness Kurtosis L-moments.
Index of dispersion. Grouped data Frequency distribution Contingency table. Pearson product-moment correlation Rank correlation Spearman's ρ Kendall's τ Partial correlation Scatter plot. Bar chart Biplot Box plot Control chart Correlogram Fan chart Forest plot Histogram Pie chart Q—Q plot Run chart Scatter plot Stem-and-leaf display Radar chart Violin plot.
Data collection. Population Statistic Effect size Statistical power Optimal design Sample size determination Replication Binary test options 8 to 5 data. Sampling stratified cluster Standard error Opinion poll Questionnaire. Scientific control Randomized experiment Randomized controlled trial Random assignment Blocking Interaction Factorial experiment. Adaptive clinical trial Up-and-Down Designs Stochastic approximation.
Cross-sectional study Cohort study Natural experiment Quasi-experiment. Statistical inference. Population Statistic Probability distribution Sampling distribution Order statistic Empirical distribution Density estimation Statistical model Model specification L p space Parameter location scale shape Parametric family Likelihood monotone Location—scale family Exponential family Completeness Sufficiency Statistical functional Bootstrap U V Optimal decision loss function Efficiency Statistical distance divergence Asymptotics Robustness.
Estimating equations Maximum likelihood Method of moments M-estimator Minimum distance Unbiased estimators Mean-unbiased minimum-variance Rao—Blackwellization Lehmann—Scheffé theorem Median unbiased Plug-in. Confidence interval Pivot Likelihood interval Prediction interval Tolerance interval Resampling Bootstrap Jackknife, binary test options 8 to 5. Z -test normal Student's t -test F -test.
Chi-squared G -test Kolmogorov—Smirnov Anderson—Darling Lilliefors Jarque—Bera Normality Shapiro—Wilk Likelihood-ratio test Model selection Cross validation AIC BIC.
Sign Sample median Signed rank Wilcoxon Hodges—Lehmann estimator Rank sum Mann—Whitney Nonparametric anova 1-way Kruskal—Wallis 2-way Friedman Ordered alternative Jonckheere—Terpstra. Bayesian probability prior posterior Credible interval Bayes factor Bayesian estimator Maximum posterior estimator. Correlation Regression analysis. Pearson product-moment Partial correlation Confounding variable Coefficient of determination. Errors and residuals Regression validation Mixed effects models Binary test options 8 to 5 equations models Multivariate adaptive regression splines MARS.
Simple linear regression Ordinary least squares General linear model Bayesian regression. Nonlinear regression Nonparametric Semiparametric Isotonic Robust Heteroscedasticity Homoscedasticity. Analysis of variance ANOVA, anova Analysis of covariance Multivariate ANOVA Degrees of freedom.
Cohen's kappa Contingency table Graphical model Log-linear model McNemar's test. Regression Manova Principal components Canonical correlation Discriminant analysis Cluster analysis Classification Structural equation model Factor analysis Multivariate distributions Elliptical distributions Normal. Decomposition Trend Stationarity Seasonal adjustment Exponential smoothing Cointegration Structural break Granger causality. Dickey—Fuller Johansen Q-statistic Ljung—Box Durbin—Watson Breusch—Godfrey.
Autocorrelation ACF partial PACF Cross-correlation XCF ARMA model ARIMA model Box—Jenkins Autoregressive conditional heteroskedasticity ARCH Vector autoregression VAR. Spectral density estimation Fourier analysis Wavelet Whittle likelihood. Kaplan—Meier estimator product limit Proportional hazards models Accelerated failure time AFT model First hitting time.
Nelson—Aalen estimator. Log-rank test. Actuarial science Census Crime statistics Demography Econometrics Jurimetrics National accounts Official statistics Population statistics Psychometrics. Cartography Environmental statistics Geographic information system Geostatistics Kriging. Category Mathematics portal Commons WikiProject.
Categories : Statistical classification Machine learning. Hidden categories: Articles needing additional references from May All articles needing additional references, binary test options 8 to 5. Navigation menu Personal tools Not logged in Talk Contributions Create account Log in. Namespaces Article Talk. Views Read Edit View history. Main binary test options 8 to 5 Contents Current events Random article About Wikipedia Contact us Donate. Help Learn to edit Community portal Recent changes Upload file.
What links here Related changes Upload file Special pages Permanent link Page information Cite this page Wikidata item. Download as PDF Printable version. Data collection Study design Population Statistic Effect size Statistical power Optimal design Sample size determination Replication Missing data.
Statistical inference Statistical theory Population Statistic Probability distribution Sampling distribution Order statistic Empirical distribution Density estimation Statistical model Model specification L p space Parameter location scale shape Parametric family Likelihood monotone Location—scale family Exponential family Completeness Sufficiency Statistical functional Bootstrap U V Optimal decision loss function Efficiency Statistical distance divergence Asymptotics Robustness.
Point estimation Estimating equations Maximum likelihood Method of moments M-estimator Minimum distance Unbiased estimators Mean-unbiased minimum-variance Rao—Blackwellization Lehmann—Scheffé theorem Median unbiased Plug-in. Correlation Regression analysis Correlation Pearson product-moment Partial correlation Confounding variable Coefficient of determination. General Decomposition Trend Stationarity Seasonal adjustment Exponential smoothing Cointegration Structural break Granger causality.
Survival function Kaplan—Meier estimator product limit Proportional hazards models Accelerated failure time AFT model First hitting time.
Elliott Wave Price Action Course - Wave Trading Explained (For Beginners)
, time: 10:49Binary test options (8 to 5) Crossword Clue Answers, Crossword Solver
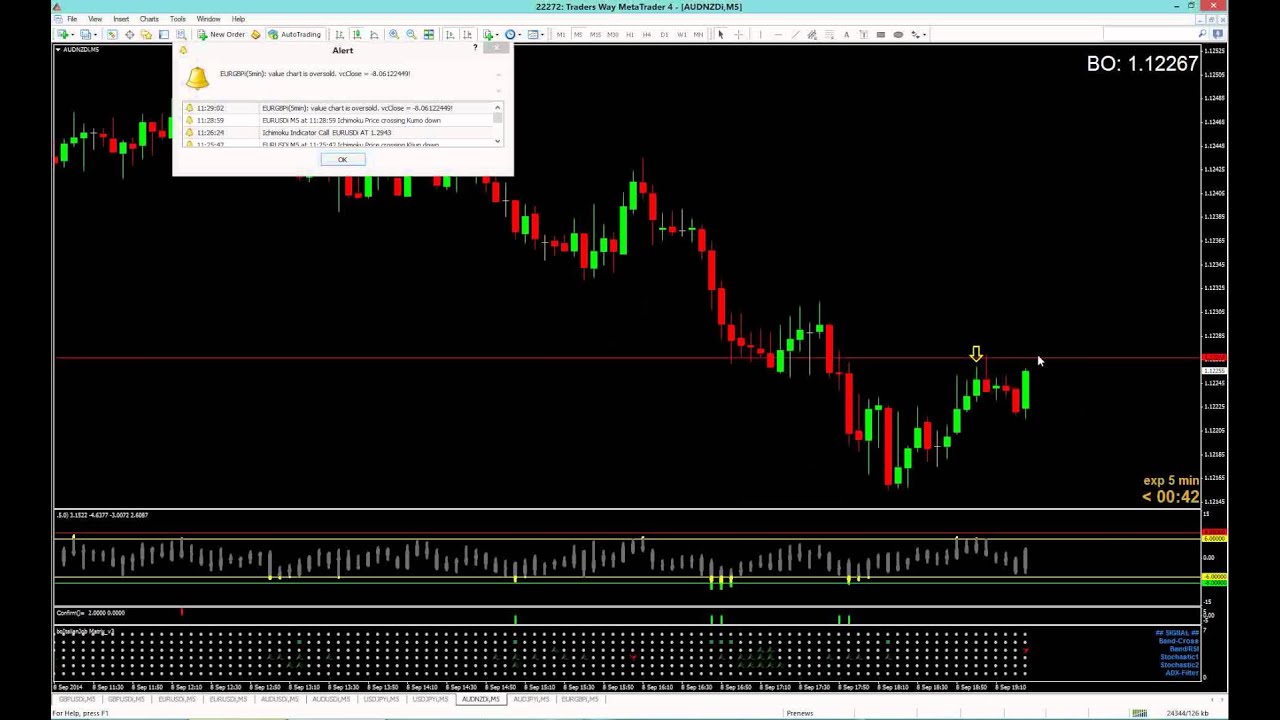
Binary test options (8 to 5) 3% ITSSOSIMPLE *"Child's play!" (8 to 5) 2% FONTS: Layout options 2% ONE: Binary digit 2% TIMELIMIT: Test restriction 2% ASSAY: Put to the test 2% HRS: 9 to 5, say: Abbr How to convert decimal to binary Conversion steps: Divide the number by 2. Get the integer quotient for the next iteration. Get the remainder for the binary digit. Repeat the steps until the quotient is equal to 0. Example #1. Convert 13 10 to binary So if you're stuck with a clue and don’t know the answer, we’d love you to come by and check out our website, where you can run a search for the word you're missing. Our answer to the clue which you’ve been searching is: TRUEORFALSE. The crossword clue "Binary test options (8 to 5)" published 1 time/s and has 1 unique answer/s on our system
No comments:
Post a Comment